The vast majority of systems that you build will inevitably call a HTTP API at some point. Whether it's a microservice, a third party API, or a legacy system. Because of this, it's not uncommon to see applications with reams of configuration variables defining where their downstream dependencies live.
This configuration is frequently a source of pain and duplication, especially in larger systems where tens or hundreds of components need to keep track of location of downstream dependencies, many of which are shared, and almost all of which change depending on deployment environment.
These configuration values get everywhere in your codebases, and often are very difficult to coordinate changes to when something changes in your deployed infrastructure.
Service discovery to the rescue
Service Discovery is a pattern that aims to solve this problem by providing a centralised location for services to register themselves, and for clients to query to find out where they are. This is a common pattern in distributed systems, and is used by many large scale systems, including Netflix, Google, and Amazon.
Service registries are often implemented as a HTTP API, or via DNS records on platforms like Kubernetes.
Service discovery is a very simple pattern consisting of:
- A service registry, which is a database of services and their locations
- A client, which queries the registry to find out where a service is
- Optionally, a push mechanism, which allows services to notify clients of changes
In most distributed systems, teams tend to use infrastructure as code to manage their deployments. This gives us a useful hook, because we can use the same infrastructure as code to register services with the registry as we deploy the infrastructure to run them.
Service discovery in .NET8 and .NET Aspire
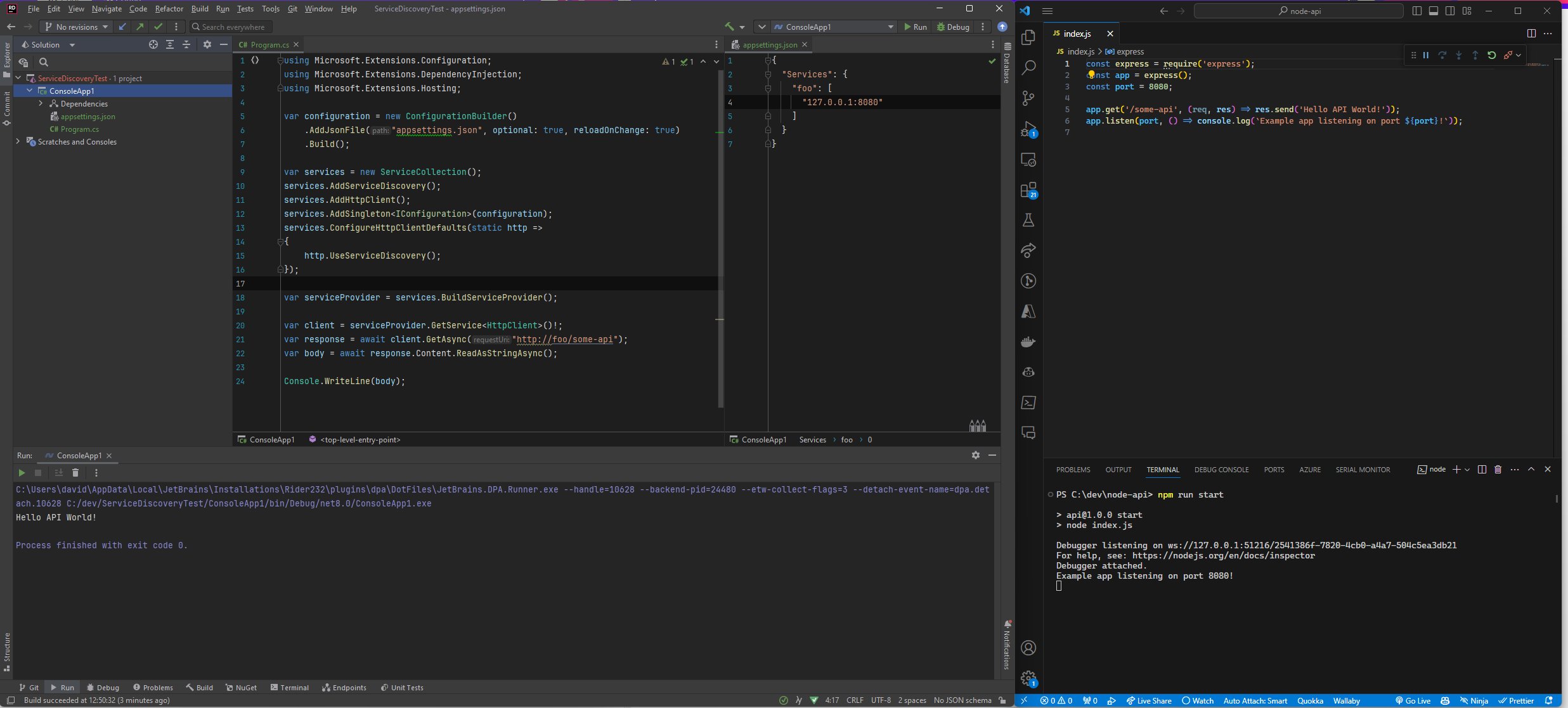
.NET 8 introduces a new extensions package - Microsoft.Extensions.ServiceDiscovery
- which is designed to interoperate with .NET Aspire, Kubernetes DNS, and App Config driven service discovery.
This package provider a hook to load service URIs from App Configuration json files, and subsequently to auto-configure HttpClient instances to use these service URIs. This allows you to use service names in the HTTP calls in your code, and have them automatically resolved to the correct URI at runtime.
This means that if you're trying to call your foo
API, that instead of calling
var response = await client.GetAsync("http://192.168.0.45/some-api");
You can call
var response = await client.GetAsync("http://foo/some-api");
And the runtime will automatically resolve the service name foo
to the correct IP address and port.
This runtime resolution is designed to work with the new Aspire stack, which manages references between different running applications to make them easier to debug, but because it has fallback hooks to App Configuration which means it can be used with anything that can load configuration settings.
Here's an example of a console application in C# 8 that uses these new service discovery features:
using Microsoft.Extensions.Configuration;
using Microsoft.Extensions.DependencyInjection;
using Microsoft.Extensions.Hosting;
// Register your appsettings.json config file
var configuration = new ConfigurationBuilder()
.AddJsonFile("appsettings.json", optional: true, reloadOnChange: true)
.Build();
// Create a service provider registering the service discovery and HttpClient extensions
var provider = new ServiceCollection()
.AddServiceDiscovery()
.AddHttpClient()
.AddSingleton<IConfiguration>(configuration)
.ConfigureHttpClientDefaults(static http =>
{
// Configure the HttpClient to use service discovery
http.UseServiceDiscovery();
})
.BuildServiceProvider();
// Grab a new client from the service provider
var client = provider.GetService<HttpClient>()!;
// Call an API called `foo` using service discovery
var response = await client.GetAsync("http://foo/some-api");
var body = await response.Content.ReadAsStringAsync();
Console.WriteLine(body);
If we pair this with a configuration file that looks like this:
{
"Services": {
"foo": [
"127.0.0.1:8080"
]
}
}
At runtime, when we make our API call to http://foo/some-api
, the HttpClient will automatically resolve the service name foo
to 127.0.0.1:8080
. For the sake of this example, we've stood up a Node/Express API on port 8080. It's code looks like this:
const express = require('express');
const app = express();
const port = 8080;
app.get('/some-api', (req, res) => res.send('Hello API World!'));
app.listen(port, () => console.log(`Example app listening on port ${port}!`));
So now, when we run our application, we get the following output:
$ dotnet run
Hello API World!
That alone is pretty neat - it gives us a single well known location to keep track of our services, and allows us to use service names in our code, rather than having to hard code IP addresses and ports. But this gets even more powerful when we combine it with a mechanism to update the configuration settings the application reads from at runtime.
Using Azure App Configuration Services as a service registry
Azure App Configuration Services provides a centralised location for configuration data. It's a fully managed service, and consists of Containers
- a key/value stores that can be used to store configuration data.
App Configuration provides a REST API that can be used to read and write configuration data, along with SDKs and command line tools to update values in the store.
When you're using .NET to build services, you can use the Microsoft.Extensions.Configuration.AzureAppConfiguration
package to read configuration data from App Configuration. This package provides a way to read configuration data from App Configuration Services, integrating neatly with the IConfiguration
API and ConfigurationManager
class.
If you're following the thread, this means that if we enable service discovery using the new Microsoft.Extensions.ServiceDiscovery
package, we can use our app config files as a service registry. If we combine this extension with Azure App Configuration Services and it's SDK, we can change one centralised configuration store and push updates to all of our services whenever changes are made.
This is really awesome, because it means if you're running large distributed teams, so long as all the applications have access to the configuration container, they can address each other by service name, and the service discovery will automatically resolve the correct IP address and port, regardless of environment.
Setting up Azure App Configuration Services
You'll need to create an App Configuration Service. You can do this by going to the Azure Portal, and clicking the "Create a resource" button. Search for "App Configuration" and click "Create".
For the sake of this example, we're going to grab a connection string from the portal, and use it to connect to the service. You can do this by clicking on the "Access Keys" button in the left hand menu, and copying the "Primary Connection String". You'd want to use RBAC in a real system.
We're going to add an override by clicking "Configuration Explorer" in the left hand menu, and adding a new key called Services:foo
with a value of:
[
"value-from-app-config:8080"
]
and a content type of application/json
.
Setting up the Azure App Configuration SDK
We need to add a reference to the Microsoft.Extensions.Configuration.AzureAppConfiguration
package to access this new override. You can do this by running the following command in your project directory:
dotnet add package Microsoft.Extensions.Configuration.AzureAppConfiguration
Next, we modify the configuration bootstrapping code in our command line app.
using Microsoft.Extensions.Configuration;
using Microsoft.Extensions.DependencyInjection;
using Microsoft.Extensions.Hosting;
var appConfigConnectionString = "YOUR APP CONFIG CONNECTION STRING HERE";
var configuration = new ConfigurationBuilder()
.AddJsonFile("appsettings.json", optional: true, reloadOnChange: true)
.AddAzureAppConfiguration(appConfigConnectionString, false) // THIS LINE HAS BEEN ADDED
.Build();
This adds our Azure App Configuration as a configuration provider.
Nothing else in our calling code needs to change - so when we execute our application, you'll notice that the call now fails:
$ dotnet run
Unhandled exception. System.Net.Http.HttpRequestException: No such host is known. (value-from-app-config:8080)
---> System.Net.Sockets.SocketException (11001): No such host is known.
at System.Net.Sockets.Socket.AwaitableSocketAsyncEventArgs.ThrowException(SocketError error, CancellationToken cancellationToken)
at System.Net.Sockets.Socket.AwaitableSocketAsyncEventArgs.System.Threading.Tasks.Sources.IValueTaskSource.GetResult(Int16 token)
at System.Net.Sockets.Socket.<ConnectAsync>g__WaitForConnectWithCancellation|285_0(AwaitableSocketAsyncEventArgs saea, ValueTask connectTask, CancellationToken cancellationToken)
at System.Net.Http.HttpConnectionPool.ConnectToTcpHostAsync(String host, Int32 port, HttpRequestMessage initialRequest, Boolean async, CancellationToken cancellationToken)
--- End of inner exception stack trace ---
...
If you look at the error message carefully it's now trying to connect to value-from-app-config:8080
- which is the value we put in our App Configuration container.
In a real-world scenario, you would want to configure refreshing of the configuration values following the guides available here.
Populating values in the real world
We've gone into detail about how you can configure service discovery using a combination of the new Microsoft.Extensions.ServiceDiscovery
package, and the Microsoft.Extensions.Configuration.AzureAppConfiguration
package, along with an Azure App Configuration Service Container - but this is all useless if you can't populate the values in the first place.
Unfortunately this entirely depends on how you automate your deployments. But in principle, you can use the Azure App Configuration SDK or API to populate the values in the container. You'll likely want to do this when your infrastructure as code runs (Pulumi/Bicep/Terraform), or as part of your CI/CD pipeline.
As part of these updates, I'd also recommend hasing all the values and adding a checksum key into the configuration store. This will allow you to monitor a single key from the client side SDKs, and trigger a refresh when the checksum changes.
If you're still working on automating your infrastructure, this technique can still be useful as you can use the portal itself to update the values in the container, and the SDK will automatically pick up the changes.
Conclusion
While a lot of the experiences being built for Aspire have limited value for larger distributed systems, I think this is an excellent example of how we can use some of the low-level features of the Aspire stack to build useful tools for other use-cases.
While we've focused purely on config file driven service discovery in this piece, you can implement custom resolvers to use other service registries like Hashicorp Consul, or another home grown solution.